- Registration deadline for the workshop: 2024-11-25 23:59:59+03:30
Keynote Speakers
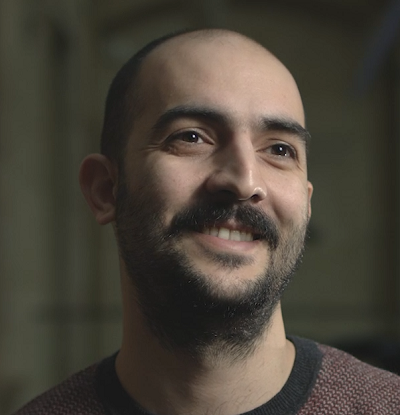
- Siavash Arjomand Bigdeli
- Associate Professor
- Technical University of Denmark - Google-Scholar
- Lecture title: Adapting AI: Explainability and Distributability
- Lecturer biography:
-
Siavash is a tenure-track associate professor for deep learning and computer vision at DTU, Visual Computing Section. Before that, he was a postdoctoral researcher at EPFL, hosted by Professor Sabine Süsstrunk. He obtained a Ph.D. from University of Bern under the supervision of Professor Matthias Zwicker. Prior to joining DTU, he was a senior scientist in CSEM.
He is interested in machine learning to build explicit and explainable statistical models for Computer Vision and Graphics applications. - Location: Online presentation in AUT Central Amphitheater Hall, or can participate via https://bluemeet.aut.ac.ir/ch/aaic_lectures/guest from anywhere. The lecture is open to everyone.
- Date: Nov 27 2024, 11:00:00
Mainstream AI development embraces autonomous system decisions. While this technology has significant applications and benefits, this global trend may be limiting to people's epistemic freedom and access to tools. Through a brief examination of epistemic and accessibility concerns in Iran, we review examples that highlight such limitations and discuss how existing AI tools fail to meet these needs. Based on this analysis, we propose a novel AI approach with explainable decision-making and distributable access through networks.
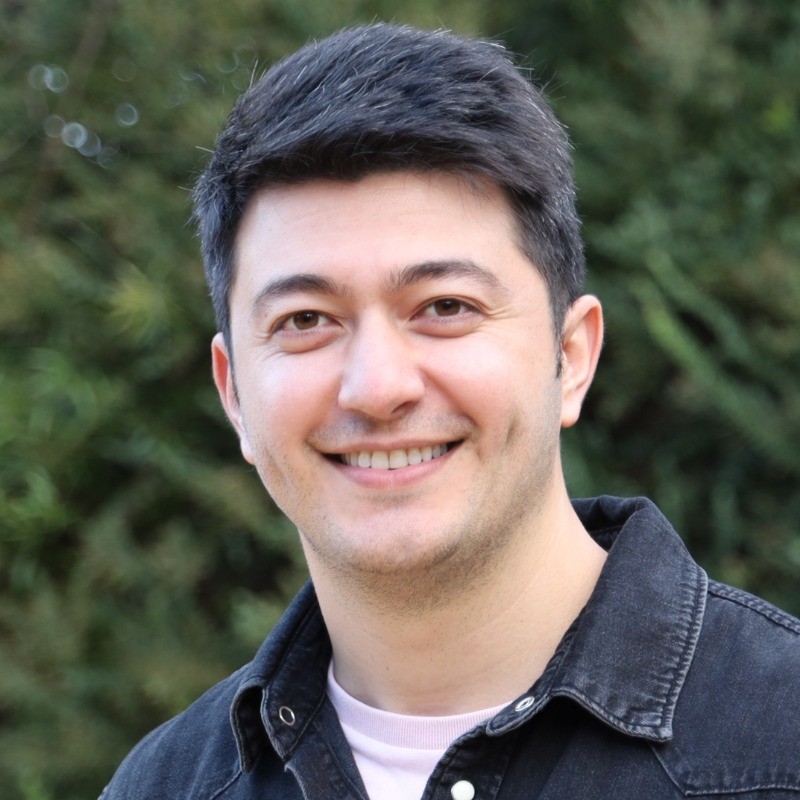
- Navid Mohammadi Foumani
- Ph.D.
- Monash University - Google-Scholar
- Lecture title: Tackling Challenges in Self-supervised EEG Representation Learning
- Lecturer biography:
-
AI/ML Specialist@Emotiv
- Location: Online presentation in AUT Central Amphitheater Hall, or can participate via https://bluemeet.aut.ac.ir/ch/aaic_lectures/guest from anywhere. The lecture is open to everyone.
- Date: Nov 27 2024, 13:00:00
Electroencephalography (EEG) is a technique that records the brain's electrical activity, offering insights into mental health and aiding in diagnosing conditions like sleep disorders, epilepsy, and more. However, analyzing EEG data is challenging due to noise, wide variability in signal strengths, and its continuous nature. Our approach, EEG2Rep, introduces a new way of learning meaningful patterns from EEG without needing labeled data. Instead of focusing on raw EEG signals prone to noise, EEG2Rep works in a "latent space" to extract cleaner, more useful features. Additionally, it uses a novel method to preserve meaningful patterns during training, resulting in better and more reliable representations. EEG2Rep has shown outstanding performance across multiple tasks, surpassing existing methods. This work paves the way for improvements in healthcare and brain research by enabling more efficient and accurate EEG analysis.
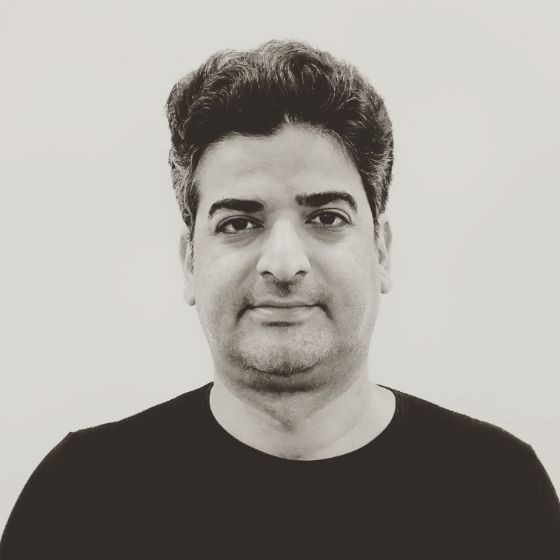
- Mohammad Sabokrou
- Ph.D. - Staff Research Scientist
- Okinawa Institute of Science and Technology (OIST) - Google-Scholar
- Lecture title: Universal Novelty Detection
- Lecturer biography:
-
Staff Scientist at OIST
- Location: Oral presentation in AUT Central Amphitheater Hall
- Date: Nov 27 2024, 14:00:00
This talk focuses on the critical task of novelty detection for deploying machine learning models in real-world scenarios. A key aspect of novelty detection methods is their universality, or their ability to generalize across various distributions of training and test data. Distribution shifts can occur in either the training or the test set. Training set shifts involve training a novelty detector on a new dataset and expecting strong transferability, while test set shifts pertain to the method's performance when encountering a shifted test sample.
Our experimental results reveal that existing methods struggle to maintain universality due to their rigid inductive biases. To address this, we aim to develop more generalized techniques with adaptable inductive biases. By leveraging contrastive learning, we create an efficient framework to switch and adapt to new inductive biases through appropriate augmentations in forming negative pairs.
We introduce a novel probabilistic auto-negative pair generation method, AutoAugOOD, combined with contrastive learning to establish a universal novelty detection method.
This talk is based on our findings from two papers:
Universal Novelty Detection Through Adaptive Contrastive Learning (https://openaccess.thecvf.com/content/CVPR2024/papers/Mirzaei_Universal_Novelty_Detection_Through_Adaptive_Contrastive_Learning_CVPR_2024_paper.pdf), CVPR2024 and Enhancing Anomaly Detection Generalization through Knowledge Exposure: The Dual Effects of Augmentation (https://arxiv.org/pdf/2406.10617).